The Converging Frontiers of AI and Oculomics: A review for eye care and AHPs
Introduction
The eye as a “window” to overall health is a long-established concept, dating from millenia ago.1 Historically, not only have signs like jaundice or hypertensive retinopathy been indications of systemic conditions, but even ancient diseases (for example Tuberculosis, which Greek physician Hippocrates described as causing “sparkling eyes”).
This awareness of the eye-systemic link has increased rapidly over recent decades. This has accelerated with technological advancements, leading to the term “Oculomics”—using ophthalmic biomarkers for systemic insights. The advent of Big Data, and Artificial Intelligence (AI), particularly machine learning (ML) and deep learning (DL), have provided powerful tools to analyse complex ophthalmic data, and AI algorithms detect subtle patterns imperceptible to humans, correlating them with systemic conditions before symptoms appear.
This promises earlier disease detection and risk stratification.2 The objective will be the enhancement of preventative care (particularly given the accessibility of community optometry), with the field set to have applications across primary care, specialised clinics, and particularly low-resource settings.
This review provides a key overview of AI and Oculomics for allied health professionals and covers the science linking ocular biomarkers to systemic health, AI techniques, relevant diseases, current tools, practical implications, challenges (technical, ethical, regulatory), and future directions, aiming to enhance interdisciplinary care.
Oculomics: The Eye as a Window to Systemic Health
The core principle within Oculomics is that measurable ocular changes can reflect systemic pathological processes. 3
Scientific Basis for the Eye-Systemic Link
The validity of oculomics rests principally on the following key biological connections:
- Shared Vasculature: The eye allows non-invasive observation of the microcirculation. Retinal vessels share similarities with those in the heart, brain, and kidneys.
- Shared Neural Tissue: The retina and optic nerve are extensions of the central nervous system (CNS).
- Immune and Metabolic Connections: Systemic inflammation and autoimmune conditions (e.g., Rheumatoid Arthritis, Sjögren’s Syndrome) can manifest as ocular inflammatory disease4
Key Enabling Imaging Modalities
Although not exhaustive, much of the insights into the Oculome are arising due to the array of advanced non-invasive imaging we have:5
- Optical Coherence Tomography (OCT): Provides high-resolution, cross-sectional retinal images (“optical biopsy”), enabling precise measurement of RNFL and GCL thickness, crucial for neurodegenerative diseases in particular, with SD-OCT and SS-OCT offering improved resolution and speed.
- OCT Angiography (OCTA): Visualizes retinal/choroidal blood flow without dye, quantifying vessel density, perfusion, and foveal avascular zone (FAZ) size. This is valuable for assessing subtle microvascular changes in diabetes, hypertension, CVD, and neurodegenerative conditions.
- Color Fundus Photography (CFP): Standard 2D imaging which allows quantitative analysis of vessel calibre/tortuosity for cardiovascular risk.
- Other Technologies: Ultra-widefield (UWF) imaging, corneal confocal microscopy (IVCM), fundus autofluorescence, spectroscopy, hyperspectral imaging, and visible-light OCT are all expanding biomarker detection.
Oculomics does not just rely on imaging: Non-image ocular biomarkers, for example tear proteins or molecular components within ocular fluids and tissues, or oculometrics (for example, eye movements), visual fields and orthoptic assessments also fit within this umbrella.
Artificial Intelligence Techniques Powering Oculomic Analysis
AI in Ophthalmology Overview
Ophthalmology is heavily impacted by AI due to its dependence on imaging modalities generating vast digital data. AI, particularly deep learning, excels at analysing these images for automated diagnosis (e.g., DR, AMD, glaucoma) and extracting oculomic biomarkers, and may even match or exceed human expert performance in specific tasks like DR detection.6
Key AI Glossary
- Machine Learning (ML): Algorithms learn patterns from data (ocular images + systemic health info) to make predictions without explicit programming. This can analyse extracted features or raw data.
- Deep Learning (DL): The application of multi-layered neural networks to automatically learn hierarchical data representations from raw images, bypassing manual feature engineering
- Computer Vision: The broader field enabling computers to understand images, using AI techniques like ML/DL.
- Other AI Approaches: These include: Traditional ML (e.g., SVMs) for feature-based prediction; Transformers for image analysis; Natural Language Processing (NLP) for clinical notes.
When applied to colour fundus photographs, the above have enabled screening and grading for diabetic retinopathy (DR), detection of age-related macular degeneration (AMD) and glaucoma, together with prediction of systemic variables like age, sex, blood pressure, HbA1c, and cardiovascular disease risk. With Optical Coherence Tomography (OCT) scans, AI detects and quantifies macular oedema, segments retinal layers for neurological and glaucoma assessment, classifies AMD, and identifies fluid. Furthermore, AI can analyse OCT Angiography (OCTA) scans to quantify microvascular parameters such as vessel density and foveal avascular zone (FAZ) area, which are linked to DR, AMD, glaucoma, and numerous systemic vascular or neurodegenerative diseases.
This harmony between AI and imaging allows for the extraction of subtle patterns often missed by humans, demonstrating “super-human” predictive capabilities for systemic conditions directly from eye images and uncovering novel correlations. Future developments aim towards multimodal AI, integrating imaging data with electronic health records (EHRs) and genomics, allowing for more comprehensive patient assessments.
Systemic Diseases with Detectable Ocular Biomarkers
The application of AI to detect ocular signs has been explored in the following systemic diseases7:
- Cardiovascular Diseases (CVD)
- Neurological Disorders
- Autoimmune Conditions
- Chronic Kidney Disease (CKD)8
- Diabetes Mellitus (DM)
This breadth of conditions within the eye reinforces its role as a systemic health indicator, symbolised in antiquity by the Eye of Horus1. However, while some signs are specific, many (e.g., vessel calibre changes, RNFL thinning) reflect common underlying processes such as microvascular damage or neurodegeneration, and the interpretation often requires clinical context.
Table 1: Ocular Manifestations of Key Systemic Diseases Detectable via Oculomics – a concise summary:
(Note: This table provides illustrative examples. Manifestations can overlap, and multiple imaging modalities may be used. AI applications are rapidly evolving and the applications may become out-of-date swiftly.
Systemic Disease Category | Specific Disease(s) Example | Key Ocular Biomarkers/Signs | Primary Imaging Modality (for Oculomics) | AI Application Example (if applicable) |
Cardiovascular | Hypertension, Atherosclerosis, Heart Failure | Retinal vessel calibre changes (narrow arterioles, wide venules), AV nicking, haemorrhages, cotton wool spots, RVO/RAO, reduced vessel density, enlarged FAZ | CFP, OCTA | AI prediction of BP, CVD risk, MACE from CFP |
Diabetes Mellitus | Type 1 & Type 2 Diabetes | Microaneurysms, haemorrhages, exudates, neovascularization (DR), macular edema (DME), reduced capillary density, FAZ changes, choriocapillaris flow deficits | CFP, OCT, OCTA | Autonomous DR screening (IDx-DR, EyeArt) |
Neurological | Alzheimer’s Disease, Parkinson’s Disease, Multiple Sclerosis | RNFL thinning, GCL thinning, reduced macular volume, optic nerve changes, altered vessel density/tortuosity, corneal nerve changes | OCT, OCTA, Corneal Confocal Microscopy | AI detection of preclinical PD , AD risk prediction |
Autoimmune | Sjögren’s, Rheumatoid Arthritis, SLE, Spondylitis | Keratoconjunctivitis Sicca (Dry Eye), Uveitis, Scleritis/Episcleritis, Retinal Vasculitis, Optic Neuritis, Corneal thinning/keratitis | Slit Lamp Exam, CFP, OCT (for uveitis complications) | AI less established for primary diagnosis, may aid in monitoring complications |
Chronic Kidney Disease | CKD (various stages) | Hypertensive/Diabetic retinopathy signs, potentially specific vessel changes, increased prevalence of cataract, AMD, glaucoma risk | CFP, OCT, OCTA | AI prediction of CKD status/risk from CFP |
Current AI-Powered Diagnostic Tools
This is a non-exhaustive summary of current, clinically-validated, AI-power diagnostic tools which have had applications within Oculomics.
Specific Platforms & Algorithms:
- IDx-DR / LumineticsCore (Digital Diagnostics)
- EyeArt (Eyenuk)
- AEYE-DS (AEYE Health)
- Google AI / Verily / DeepMind: Influential research demonstrating DL for DR, AMD, glaucoma detection and predicting systemic factors (age, sex, BP, HbA1c, CVD risk) from fundus photos. Collaborated with Moorfields Eye Hospital.
- Other Examples
Currently, the application of these platforms has primarily been in screening – automated DR detection in primary care/community settings to improve access and efficiency. This is emergent for AMD and glaucoma, and broadening to include other ocular conditions. However the objective remains for clinical validation of risk stratification/prediction, i.e. using ocular biomarkers to predict future systemic disease risk (CVD, AD, CKD), which might include predicting physiological parameters (BP, HbA1c). And from here, diagnosis and decision support: assisting clinicians with broader diagnoses or prognostication.
The main challenges with these platforms are validation and regulatory approval.
Practical Implications and Roles in Healthcare
Benefits of AI-Oculomics Integration
This summarises the benefits of expanding the role of AI and Oculomics into healthcare systems around the world:
- Early Detection/Prevention: Identify systemic diseases earlier, allowing timely interventions.
- Increased Accessibility/Equity: Deploy screening in non-specialist settings (primary care, pharmacies), in particular to reach underserved populations.
- Efficiency/Workflow Optimization: Automate image analysis, reducing workload, speeding results, allowing clinicians to focus on complex tasks
- Non-Invasiveness/Convenience: Retinal imaging is quick, painless, and well-tolerated.
- Potential Cost-Effectiveness: Early detection/prevention may reduce long-term costs; efficient screening reduces operational costs
Roles for Healthcare Professionals
Healthcare professionals around the world are set to be involved in this revolution:
- Optometrists: Key users for enhanced screening (e.g., DR), interpreting AI outputs, identifying systemic risk indicators, counselling, and referring according to local pathways (NHS, HSE, US models).
- Ophthalmologists (inc. Trainees): Manage complex cases identified by AI, confirm diagnoses, treat, provide clinical oversight/QA for AI systems. AI education will become more of a syllabus requirement in Ophthalmology training schemes.
- Nurses (Ophthalmic, Practice, Community): Operate AI cameras, perform screening, manage patient flow, provide education, facilitate referrals and care coordination.
- Pharmacists: Potential accessible screening locations (e.g., DR for diabetics). Could link results to counselling, provide advice, and facilitate referrals.
Integration into Healthcare Systems
Assimilation of AI and Oculomics requires delivery of the following:
- Clinical Pathways: Clear protocols are needed for acting on AI results (referral criteria, timelines, responsibilities)
- EHR Integration/Interoperability: Critical for workflow and data sharing, but significant challenges remain.
- Commissioning/Reimbursement: Requires sustainable funding models (eg NHS/HSE commissioning, US insurance codes))
- Workforce Training/Change Management: Training on AI use, capabilities, limitations.
Effective integration demands integrated, multidisciplinary care, breaking down specialty silos, and implementation models will vary by healthcare system. Realising benefits requires overcoming practical hurdles like workflow integration, interoperability, training, cost, and demonstrating value.
Challenges and Considerations
Significant challenges must be addressed for safe, effective, and equitable AI-Oculomics implementation, which span technical, regulatory, and ethical areas9.
AI deployment in healthcare, including in fields like Oculomics, faces significant technical, ethical, regulatory, and adoption challenges. Technically, AI demands large volumes of high-quality, labeled data, yet faces issues with generalizability, interoperability with EHRs, and infrastructure limitations. Ethically, concerns include data privacy, algorithmic bias, lack of transparency, unclear liability, and the risk of clinician deskilling. Regulatory hurdles involve evolving approval pathways, ambiguous validation standards, and limited post-market surveillance. Clinician and patient acceptance hinges on trust, ease of integration, and clarity on roles. Overcoming these barriers requires a coordinated, multi-stakeholder effort across the healthcare ecosystem.
The Future of AI in Oculomics
The field continues to evolve rapidly – and promises a future where increasingly affordable and accessible digital health integration will lead to more personalised medicine and customised treatment strategies for patients, with patient safety underpinned throughout.
Emerging Research Areas will include:
- Novel Biomarker Discovery: AI identifying new, subtle oculomic biomarkers for systemic disease.
- Predicting Treatment Response: AI predicting individual patient response to therapies, whether ophthalmic disease (eg anti-VEGF) or systemic (eg new immunosuppressants)
- Multimodal AI and Foundation Models: Integrating multiple imaging modalities (OCT+OCTA+CFP) and non-imaging data (genomics, EHRs), with larger and more versatile “foundation models” becoming available
- Longitudinal Monitoring and Home-Based Care: AI analysing changes over time for disease progression monitoring, with potential for home-based imaging (e.g., home OCT)
Integration with Other Health Data
Deeper integration of oculomic data with EHRs, genomic datasets, wearables, etc., will become more and more prevalent, and projects like AI-READI are creating linked multimodal datasets. Data fusion will allow for integrative holistic digital patient representations and personalised predictions.
Impact on Preventative Medicine and Public Health
AI-Oculomics could transform preventative medicine by enabling population-level screening for chronic diseases (CVD, diabetes, neurodegeneration) via routine eye exams. Scalable, low-cost, non-invasive retinal imaging with AI analysis facilitates early detection and intervention, potentially reducing morbidity, mortality, and healthcare costs.
Technological Advancements
- Imaging Technology: Improvements in resolution, speed, field of view (UWF), depth (SS-OCT), and accessibility (portable/smartphone cameras) will provide richer data.
- AI Algorithms: More sophisticated, accurate, generalizable, explainable, and adaptive algorithms are expected, with afore-mentioned foundation models would provide more versatility.
- Data Infrastructure: Robust, secure, interoperable platforms for large multimodal datasets are crucial.
Translating potential into practice requires ongoing validation, addressing bias, improving explainability, and navigating ethical/regulatory pathways.
Take Home Message
The harmony between AI and oculomics marks a significant medical frontier. The eye provides a unique window to systemic health, and AI analysis of ophthalmic enables detection of biomarkers for cardiovascular, diabetic, neurodegenerative, autoimmune, and renal diseases, often early.
Potential benefits include earlier detection, increased screening accessibility, enhanced efficiency, and non-invasiveness, positioning AI-oculomics as a powerful tool for preventative medicine. Healthcare professionals throughout the world have key roles in implementation, requiring adaptation and integrated care pathways. However, technical (data quality, generalizability), ethical (privacy, bias, accountability), and regulatory challenges must be addressed.
Future directions involve sophisticated multimodal AI integrated with broader health data. Collaborative efforts are crucial to responsibly harness AI-oculomics’ transformative potential for improving global health outcomes.
References
- Ghadiri, N. AI & Oculomics: a new section for Eye News, Eye News. Available at: https://www.eyenews.uk.com/features/ai-oculomics/post/ai-oculomics-a-new-section-for-eye-news
- Oculomics: the science of using the eye to diagnose systemic health https://www.insight.hdrhub.org/post/oculomics-the-science-of-using-the-eye-to-diagnose-systemic-health
- Lucy Miller, L. D. (no date) Oculomics: a window to overall health, Association of Optometrists (AOP) – Homepage. Available at: https://www.aop.org.uk/ot/videos/2024/12/10/oculomics-a-window-to-overall-health
- Ghadiri N. The history of uveitis: from antiquity to the present day. Eye (Lond). 2025 Feb;39(3):488-491. doi: 10.1038/s41433-024-03519-x. Epub 2024 Dec 4. PMID: 39632989; PMCID: PMC11794655.
- Betzler BK et al. Oculomics: Insights into Systemic Disease through Ophthalmic Biomarkers – Frontiers, , https://www.frontiersin.org/research-topics/25501/oculomics-insights-into-systemic-disease-through-ophthalmic-biomarkers
- Wu JH and Liu TYA Application of Deep Learning to Retinal-Image-Based Oculomics for Evaluation of Systemic Health https://www.mdpi.com/2077-0383/12/1/152
- Jai K et al. Predictive and diagnostic approaches for systemic disorders using ocular assessment. https://www.frontiersin.org/journals/medicine/articles/10.3389/fmed.2024.1529861/full
- Ghadiri N et al. A triad of retinal signs in Alport syndrome: The ‘stair-case’ fovea, choroidal thinning and peripheral schisis. European Journal of Ophthalmology. 2019;29(1_suppl):10-14.
- Johnson T et al. Ethical Considerations in AI-Powered Medical Imaging: Bias, Privacy, and Accountability. https://www.researchgate.net/publication/390113780_Ethical_Considerations_in_AI-Powered_Medical_Imaging_Bias_Privacy_and_Accountability
Latest Articles
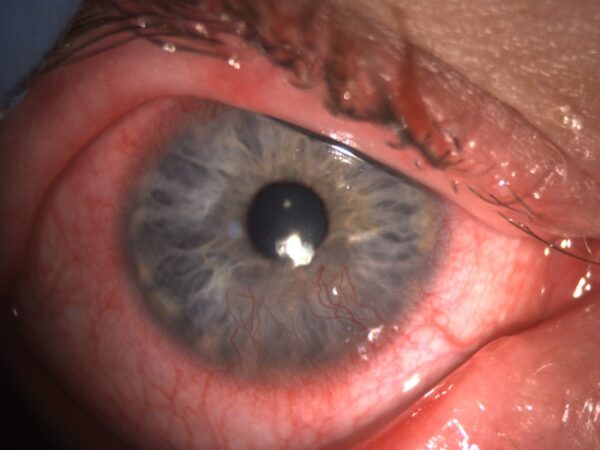
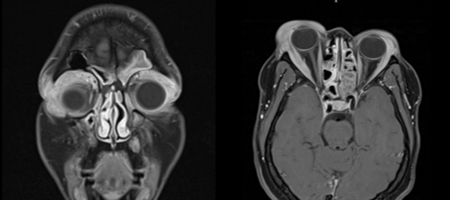
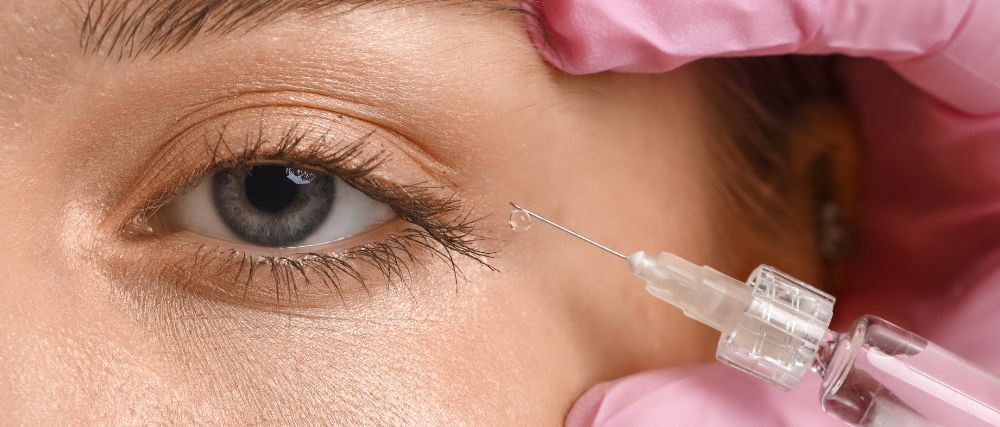
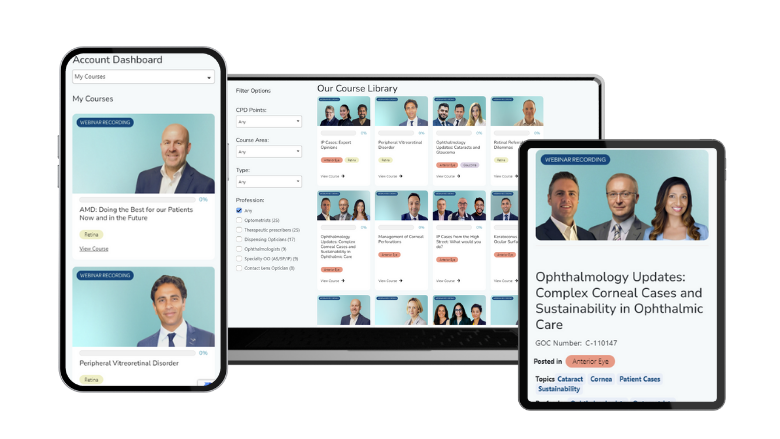
HCP Popup
Are you a healthcare or eye care professional?
The information contained on this website is provided exclusively for healthcare and eye care professionals and is not intended for patients.
Click ‘Yes’ below to confirm that you are a healthcare professional and agree to the terms of use.
If you select ‘No’, you will be redirected to scopeeyecare.com
This will close in 0 seconds